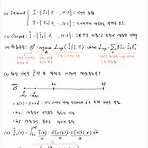
Abstract논문의 저자들은 카메라 포즈 정보 없이 NeRF를 통해 사진을 입체화하는 방법을 제안한다.1. Introduction(1) NeRF 학습의 전처리NeRF가 요구하는 전처리: COLMAP을 이용한 카메라 파라미터 추정COLMAP의 단점:처리 시간이 길다.미분 가능성(differentiability)이 없어 딥러닝과의 결합이 어렵다.(2) 카메라 포즈를 추정하는 기존의 연구연구된 모델: NeRFmm, BARF, SC-NeRF한계점: LLFF와 같은 forward-facing scene에서는 좋은 성능을 보였으나, 다이나믹한 카메라 이동에서 위치 추정 실패원인:이미지간의 상대적인 포즈를 고려하지 않고, 각 이미지마다 독립적으로 카메라 포즈를 추정함. SLAM과 Visual Odmetry는 이미지..
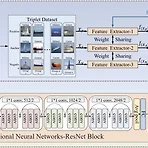
논문 제목: Addressing unfamiliar ship type recognition in real-scenario vessel monitoring: a multi-angle metric networks framework 감시 카메라로 밀입국한 중국 선박을 감지하는 인공지능 모델을 만들고 있는데, 전혀 다른 외형의 중국 어선을 few-shot learning으로 구별해낼 수 있을까 하여 해당 논문을 정리해본다. 제안된 방법론Triplet Generator각 클래스별로 분류된 선박을 Anchor, Positive, Negative의 세 이미지가 있는 묶음으로 재분할 한다. Anchor는 비교할 이미지, Positive에는 Anchor와 동일한 클래스의 이미지, Negative에는 Anchor와 다른..
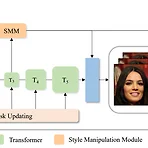
convolutional headinput data: incompleted image Im and given mask Mto extract tokensone: change the input dimensionthree: down sample the resolution(1/8 size)numbers of convolution channels and FC dimensions to 180 for the head, body, and reconstruction modules.reason of this module1. it is designed for fast downsampling to reduce computational complexity and memory cost.2. empirically f..
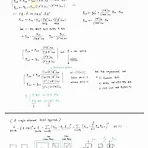
본 논문은 추천 시스템에서 Matrix Factorzation을 이용한 Collaborative Filtering 기술을 한 단계 더 진보시켰다.해당 논문을 읽으면서 필기한 내용을 정리한다.논문 링크: https://ieeexplore.ieee.org/abstract/document/6748996 An Efficient Non-Negative Matrix-Factorization-Based Approach to Collaborative Filtering for Recommender SystemsMatrix-factorization (MF)-based approaches prove to be highly accurate and scalable in addressing collaborative filteri..